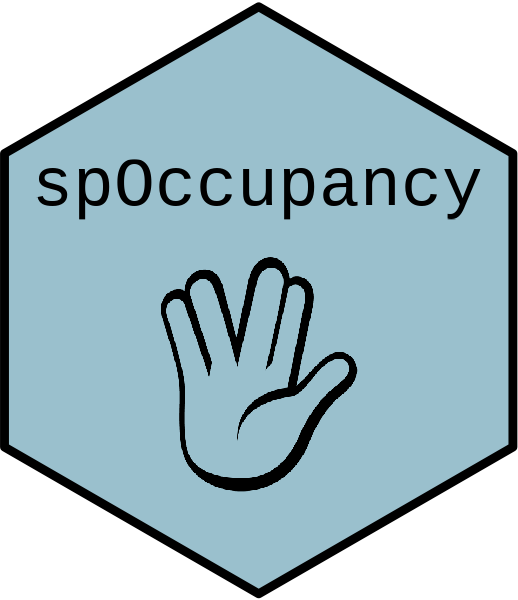
Function for prediction at new locations for single-species integrated occupancy models
predict.intPGOcc.Rd
The function predict
collects posterior predictive samples for a set of new locations given an object of class `intPGOcc`.
Usage
# S3 method for intPGOcc
predict(object, X.0, ignore.RE = FALSE, type = 'occupancy', ...)
Arguments
- object
an object of class intPGOcc
- X.0
the design matrix for prediction locations. This should include a column of 1s for the intercept. Covariates should have the same column names as those used when fitting the model with
intPGOcc
.- ignore.RE
logical value that specifies whether or not to remove random occurrence (or detection if
type = 'detection'
) effects from the subsequent predictions. IfTRUE
, random effects will be included. IfFALSE
, random effects will be set to 0 and predictions will only be generated from the fixed effects.- type
a quoted keyword indicating what type of prediction to produce. Valid keywords are 'occupancy' to predict latent occupancy probability and latent occupancy values (this is the default), or 'detection' to predict detection probability given new values of detection covariates. Note that prediction of detection probability is not currently supported for integrated models.
- ...
currently no additional arguments
Author
Jeffrey W. Doser doserjef@msu.edu,
Andrew O. Finley finleya@msu.edu
Value
An object of class predict.intPGOcc
that is a list comprised of:
- psi.0.samples
a
coda
object of posterior predictive samples for the latent occurrence probability values.- z.0.samples
a
coda
object of posterior predictive samples for the latent occurrence values.
The return object will include additional objects used for standard extractor functions.
Examples
set.seed(1008)
# Simulate Data -----------------------------------------------------------
J.x <- 10
J.y <- 10
J.all <- J.x * J.y
# Number of data sources.
n.data <- 4
# Sites for each data source.
J.obs <- sample(ceiling(0.2 * J.all):ceiling(0.5 * J.all), n.data, replace = TRUE)
# Replicates for each data source.
n.rep <- list()
for (i in 1:n.data) {
n.rep[[i]] <- sample(1:4, size = J.obs[i], replace = TRUE)
}
# Occupancy covariates
beta <- c(0.5, 1)
p.occ <- length(beta)
# Detection covariates
alpha <- list()
for (i in 1:n.data) {
alpha[[i]] <- runif(2, -1, 1)
}
p.det.long <- sapply(alpha, length)
p.det <- sum(p.det.long)
# Simulate occupancy data.
dat <- simIntOcc(n.data = n.data, J.x = J.x, J.y = J.y, J.obs = J.obs,
n.rep = n.rep, beta = beta, alpha = alpha, sp = FALSE)
y <- dat$y
X <- dat$X.obs
X.p <- dat$X.p
sites <- dat$sites
# Package all data into a list
occ.covs <- X[, 2, drop = FALSE]
colnames(occ.covs) <- c('occ.cov')
det.covs <- list()
# Add covariates one by one
det.covs[[1]] <- list(det.cov.1.1 = X.p[[1]][, , 2])
det.covs[[2]] <- list(det.cov.2.1 = X.p[[2]][, , 2])
det.covs[[3]] <- list(det.cov.3.1 = X.p[[3]][, , 2])
det.covs[[4]] <- list(det.cov.4.1 = X.p[[4]][, , 2])
data.list <- list(y = y,
occ.covs = occ.covs,
det.covs = det.covs,
sites = sites)
J <- length(dat$z.obs)
# Initial values
inits.list <- list(alpha = list(0, 0, 0, 0),
beta = 0,
z = rep(1, J))
# Priors
prior.list <- list(beta.normal = list(mean = 0, var = 2.72),
alpha.normal = list(mean = list(0, 0, 0, 0),
var = list(2.72, 2.72, 2.72, 2.72)))
# Note that this is just a test case and more iterations/chains may need to
# be run to ensure convergence.
n.samples <- 5000
out <- intPGOcc(occ.formula = ~ occ.cov,
det.formula = list(f.1 = ~ det.cov.1.1,
f.2 = ~ det.cov.2.1,
f.3 = ~ det.cov.3.1,
f.4 = ~ det.cov.4.1),
data = data.list,
inits = inits.list,
n.samples = n.samples,
priors = prior.list,
n.omp.threads = 1,
verbose = TRUE,
n.report = 1000,
n.burn = 4000,
n.thin = 1)
#> ----------------------------------------
#> Preparing to run the model
#> ----------------------------------------
#> ----------------------------------------
#> Model description
#> ----------------------------------------
#> Integrated Occupancy Model with Polya-Gamma latent
#> variable fit with 81 sites.
#>
#> Integrating 4 occupancy data sets.
#>
#> Samples per Chain: 5000
#> Burn-in: 4000
#> Thinning Rate: 1
#> Number of Chains: 1
#> Total Posterior Samples: 1000
#>
#> Source compiled with OpenMP support and model fit using 1 thread(s).
#>
#> ----------------------------------------
#> Chain 1
#> ----------------------------------------
#> Sampling ...
#> Sampled: 1000 of 5000, 20.00%
#> -------------------------------------------------
#> Sampled: 2000 of 5000, 40.00%
#> -------------------------------------------------
#> Sampled: 3000 of 5000, 60.00%
#> -------------------------------------------------
#> Sampled: 4000 of 5000, 80.00%
#> -------------------------------------------------
#> Sampled: 5000 of 5000, 100.00%
summary(out)
#>
#> Call:
#> intPGOcc(occ.formula = ~occ.cov, det.formula = list(f.1 = ~det.cov.1.1,
#> f.2 = ~det.cov.2.1, f.3 = ~det.cov.3.1, f.4 = ~det.cov.4.1),
#> data = data.list, inits = inits.list, priors = prior.list,
#> n.samples = n.samples, n.omp.threads = 1, verbose = TRUE,
#> n.report = 1000, n.burn = 4000, n.thin = 1)
#>
#> Samples per Chain: 5000
#> Burn-in: 4000
#> Thinning Rate: 1
#> Number of Chains: 1
#> Total Posterior Samples: 1000
#> Run Time (min): 0.0057
#>
#> ----------------------------------------
#> Occurrence
#> ----------------------------------------
#> Fixed Effects (logit scale):
#> Mean SD 2.5% 50% 97.5% Rhat ESS
#> (Intercept) 0.9305 0.3615 0.2652 0.9076 1.7166 NA 299
#> occ.cov 1.2033 0.3474 0.5949 1.1794 1.9201 NA 329
#>
#> ----------------------------------------
#> Data source 1 Detection
#> ----------------------------------------
#> Fixed Effects (logit scale):
#> Mean SD 2.5% 50% 97.5% Rhat ESS
#> (Intercept) -0.4199 0.3375 -1.0725 -0.4245 0.2597 NA 685
#> det.cov.1.1 -0.6578 0.4124 -1.5288 -0.6281 0.0707 NA 471
#>
#> ----------------------------------------
#> Data source 2 Detection
#> ----------------------------------------
#> Fixed Effects (logit scale):
#> Mean SD 2.5% 50% 97.5% Rhat ESS
#> (Intercept) -0.9276 0.3966 -1.7125 -0.9096 -0.1879 NA 597
#> det.cov.2.1 0.9189 0.4664 0.0876 0.9001 1.8753 NA 543
#>
#> ----------------------------------------
#> Data source 3 Detection
#> ----------------------------------------
#> Fixed Effects (logit scale):
#> Mean SD 2.5% 50% 97.5% Rhat ESS
#> (Intercept) 0.1401 0.2675 -0.3877 0.1387 0.6817 NA 667
#> det.cov.3.1 -1.0872 0.3194 -1.7360 -1.0744 -0.5203 NA 450
#>
#> ----------------------------------------
#> Data source 4 Detection
#> ----------------------------------------
#> Fixed Effects (logit scale):
#> Mean SD 2.5% 50% 97.5% Rhat ESS
#> (Intercept) -0.0591 0.3225 -0.6712 -0.0555 0.5537 NA 548
#> det.cov.4.1 0.9524 0.4511 0.1711 0.9159 1.8861 NA 609
#>
# Prediction
X.0 <- dat$X.pred
psi.0 <- dat$psi.pred
out.pred <- predict(out, X.0)
psi.hat.quants <- apply(out.pred$psi.0.samples, 2, quantile, c(0.025, 0.5, 0.975))
plot(psi.0, psi.hat.quants[2, ], pch = 19, xlab = 'True',
ylab = 'Fitted', ylim = c(min(psi.hat.quants), max(psi.hat.quants)))
segments(psi.0, psi.hat.quants[1, ], psi.0, psi.hat.quants[3, ])
lines(psi.0, psi.0)