Using autonomous monitoring systems to inform forest and wildlife management
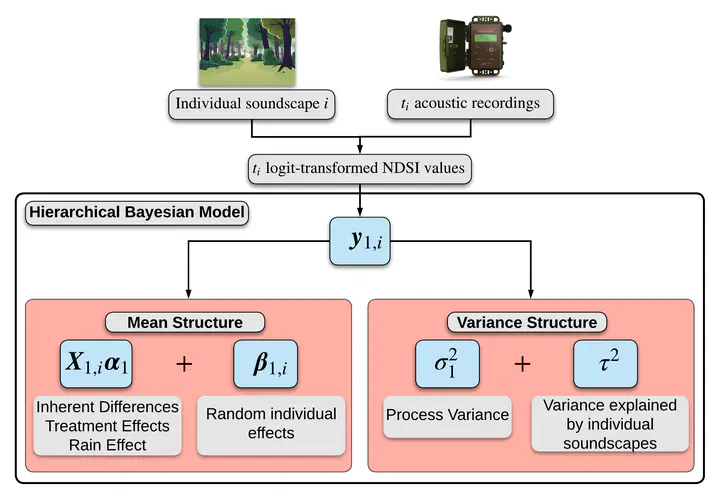
Autonomous monitoring systems such as LiDAR, acoustic recording units, camera traps, and other remote sensing methods can provide massive amounts of data to inform forest and wildlife management, yet analyzing these data presents novel computational complexities. We develop quantitative approaches to leverage these complex data to inform a variety of forest and wildlife management objectives.
A key focus in the Statistical Ecology and Forest Science lab is developing the statistical tools needed to integrate emerging technologies into existing analysis workflows to inform management objectives. Much of our work focuses on using acoustic recording units to improve insights on ecological communities. We developed hierarchical Bayesian models that leveraged acoustic recording data to understand relationships between anthropogenic noise and biological sounds in avian soundscapes (Doser et al. 2020, Landscape Ecology) and the effects of a shelterwood logging on avian soundscapes in northern Michigan (Doser et al. 2020 Ecological Indicators). Additionally, we integrated automated acoustic data, machine learning algorithms, and point count survey data in a hierarchical modeling framework to estimate bird abundance (Doser et al. 2021 MEE), which can serve as a cost-effective approach to understand spatial patterns in abundance of acoustically active species. With colleagues at the University of Montana and the National Park Service, we used automated acoustic data to inform management of Clark’s Nutcracker in relation to declining Whitebark pine trees in the western US (Kovalenko et al. 2024 Ecology and Evolution).
Future work in the Statistical Ecology and Forest Science Lab will continue to build automated workflows for the statistical analysis of acoustic data, with a particular emphasis on accounting for false positives and mis-identifications in acoustic data. Additionally, we seek to build upon existing methodological frameworks to improve the fusion of traditional forest inventory data with new remote sensing technologies like LiDAR.