Small area estimation of forest parameters
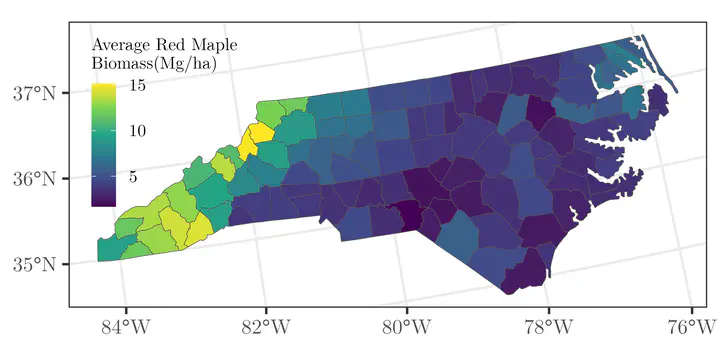
Forest management and production increasingly require estimates of forest variables in small domains. Classical design-based approaches are limited in their ability to provide unbiased estimates at small spatial scales using national forest inventory data. We develop Bayesian model-based approaches for improved estimation of forest parameters across user-defined small areas.
With collaborators at Michigan State University and the US Forest Service, we are developing multivariate spatial Bayesian models to generate improved estimates of forest parameters by species or species group. Our unit-level model-based approach shares information across space and across species groups to improve the precision of forest biomass estimates within user-defined areal regions of interest (e.g., counties, ownership blocks).
Future research in the lab will seek to developed improved methods and associated software for small area estimation (SAE) of forest parameters, in combination with regional and national stakeholders in government and the private forest sector. In particular, methodological projects include expanding the spAbundance
package and associated documentation to facilitate small area estimation using hierarchical Bayesian spatial models, a comparison of unit-level SAE models to area-level SAE models when unit level models are fit with exact spatial coordinates vs using non-exact spatial coordinates, and development of web-based applications for summarizing model results across user-defined areal units.